- Home
- Symmetry Blog
- Active Learning vs Machine Learning
Active Learning vs Machine Learning
About Jari Haiston
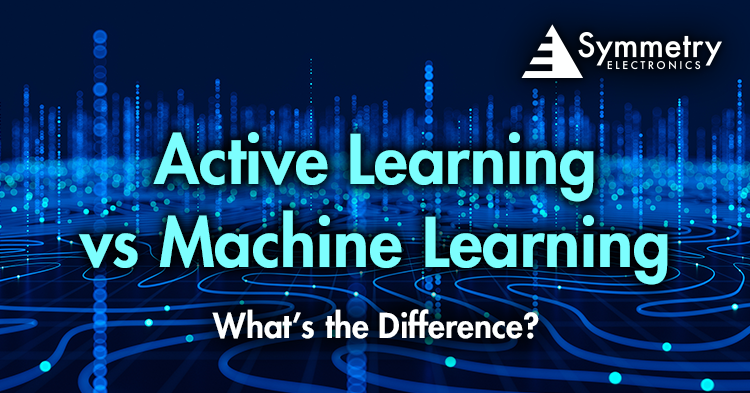
Machine learning (ML) is a pivotal branch of artificial intelligence (AI) that empowers computers to learn from data and execute tasks based on instructions. Within the realm of ML lies a specialized technique known as active learning (AL). Unlike conventional ML methods where data is passively utilized, AL involves interactive engagement, enabling a learning algorithm to solicit information from human users or other sources to label new data points with desired outputs. While both active learning and machine learning share the fundamental goal of leveraging data to make informed decisions, they differ in their approaches to acquiring and utilizing data.
Defining Active Learning and Machine Learning
Machine learning, as a subset of AI, enables computers to learn from data and perform tasks based on instruction. Active learning, on the other hand, represents a unique facet of ML, where the learning process involves interactive querying for data labeling. Though distinct, they share the fundamental goal of leveraging data to make informed decisions, highlighting the synergy between them.
In essence, AL focuses on strategies for efficient data labeling. Together, both ML and AL play integral roles in a wide range of applications. From medical diagnostics to natural language processing, their synergistic application is evident in various fields.
What is the Machine Learning Model?
An ML learning model is a program that can find patterns or make predictions from a previously unforeseen data set. An ML learning model is created from an ML algorithm, which undergoes a training process using wither labeled, unlabeled, or mixed data. There’s not one over-arching algorithm, either – different ML algorithms are suited for different goals such as classification or prediction modeling.
As a branch of AI, ML technology enables computers to learn from data and perform tasks that normally require human intelligence. ML has many applications in various fields and industries, like:
- Image Recognition
- Translation
- Fraud Detection
- Recommendation Systems
- Healthcare Diagnostics
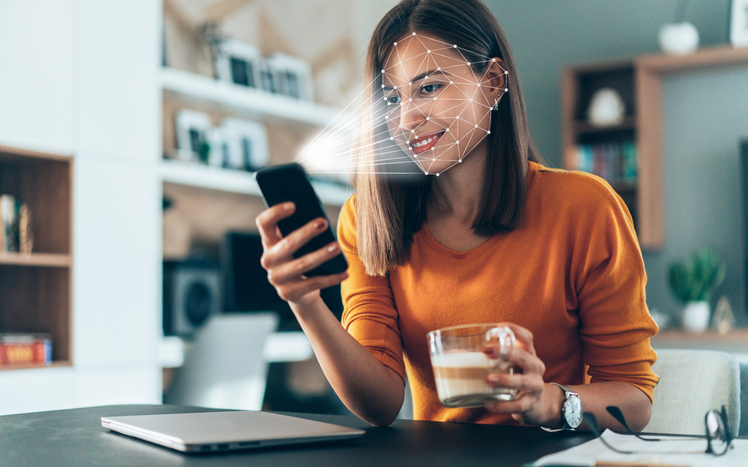
Active Learning Algorithm and Process
The entire AL process is driven by its innate benefits such as the reduction of labeling costs, enhancement of model performance, and management of over-abundant unlabeled data. Through vivid examples and illustrations, the AL process explores various query techniques, including uncertainty sampling, diversity sampling, expected error reduction, and query-by-committee.
At the heart of the AL process lies the AL algorithm, which acts as the orchestrator of data acquisition and model refinement. AL’s algorithm dynamically selects the most informative data points for labeling, optimizing learning processes by prioritizing the most beneficial instances for improving model performance. Through it iterative nature, the AL algorithm refines a model’s understanding of the data distribution, leading to more efficient and accurate learning outcomes compared to traditional passive learning approaches.
AL has proven efficacy in a diverse range of applications and use cases, like:
- Natural Language Processing
- Computer Vision
- Bioinformatics
- Recommender Systems
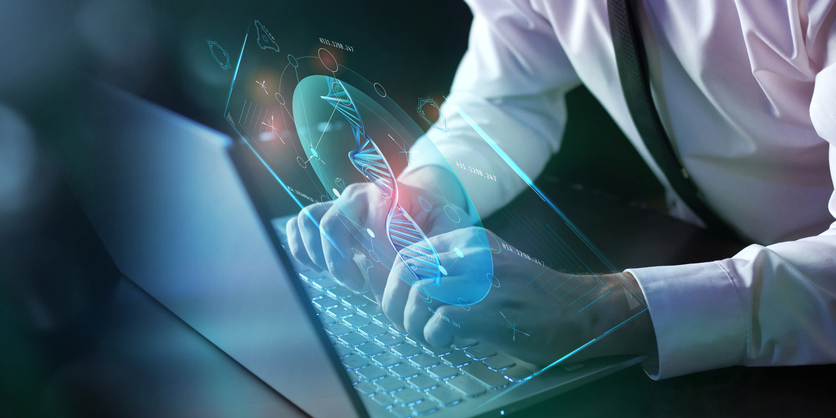
The Future of AI Learning Approaches
ML and AL technology are strategically positioned to improve the efficiency and accuracy of various processes, systems, and more. Through human effort and data reduction, ML is capable of finding difficult to obtain insights. They can also improve the generalization and robustness of models by selecting the most informative data points. ML presents personalization opportunities to create custom solutions based on user preferences. AL can be applied to enhance model transparency through human-in-the-loop feedback and adaptations.
However, AL and ML processes can be expensive and computationally complex to implement. Their integration requires designing and evaluating numerous query strategies, sampling methods, and stopping criteria. Moreover, they can be difficult to maintain once in place.
As a relatively new technology, AI learning approaches are not perfect—they can introduce biases and produce errors in data labeling processes, especially if human annotators are inconsistent, unreliable, or harmful to the overall process. ,/p>
Furthermore, ML and AL face ethical and social challenges, such as ensuring data provider privacy/consent. One of the main challenges is determining the trade-off between exploration and exploitation. That is, finding how to balance the acquisition of new information and the utilization of existing information. Another challenge is figuring out how to scale up AL processes to handle large and high-dimensional data sets, which can pose computational/memory limitations.
However, despite innate obstacles, the future is ripe with opportunities. In fact, BBC Research expects the global ML market size to grow from $17.1 billion in 2021 to a sizeable $90.1 billion in just a five-year span. The popularity of AI learning approaches can be attributed to how they enable new, innovative frameworks, streamline mundane methods, and provide users with otherwise hidden information.
AI Learning Solutions Available at Symmetry Electronics
If you’re a developer interested in designing cutting-edge solutions with AI learning integrations, Symmetry Electronics has everything you need from industry-leading suppliers to ensure your project’s success and innovation.
Digi International’s Digi ConnectCore® 93 embedded SOM is pre-certified with dual-band Wi-FI 6 and Bluetooth 5.3 connectivity. It’s designed for longevity and scalability for use in medical, industrial, energy, and transportation applications.
Integrating MediaTek’s state-of-the-art Genio 700, the Tungsten700 SMARC (smart mobility structure) Series from Laird Connectivity includes line of feature rich, high-performance SOMs and a carrier board that provides developers with all the tools necessary to create market-ready solutions.
Additionally, MediaTek’s Genio solutions include their comprehensive Genio 1200 EVK. As a high-end edge-IoT evaluation kit, the Genio 1200 is ideally suited for a broad range of applications including, industrial, smart home, interactive retail, and commercial sectors.
The xcore.ai line from XMOS assists developers in unlocking optimal performance in digital signal processing (DSP) systems. In the realm of intelligent IoT applications, xcore.ai solutions are positioned as indispensable tools to push the boundaries of what’s possible in the traditional IoT landscape.